Labeling Tools for Machine Learning: Elevating Your Data Annotation Strategy
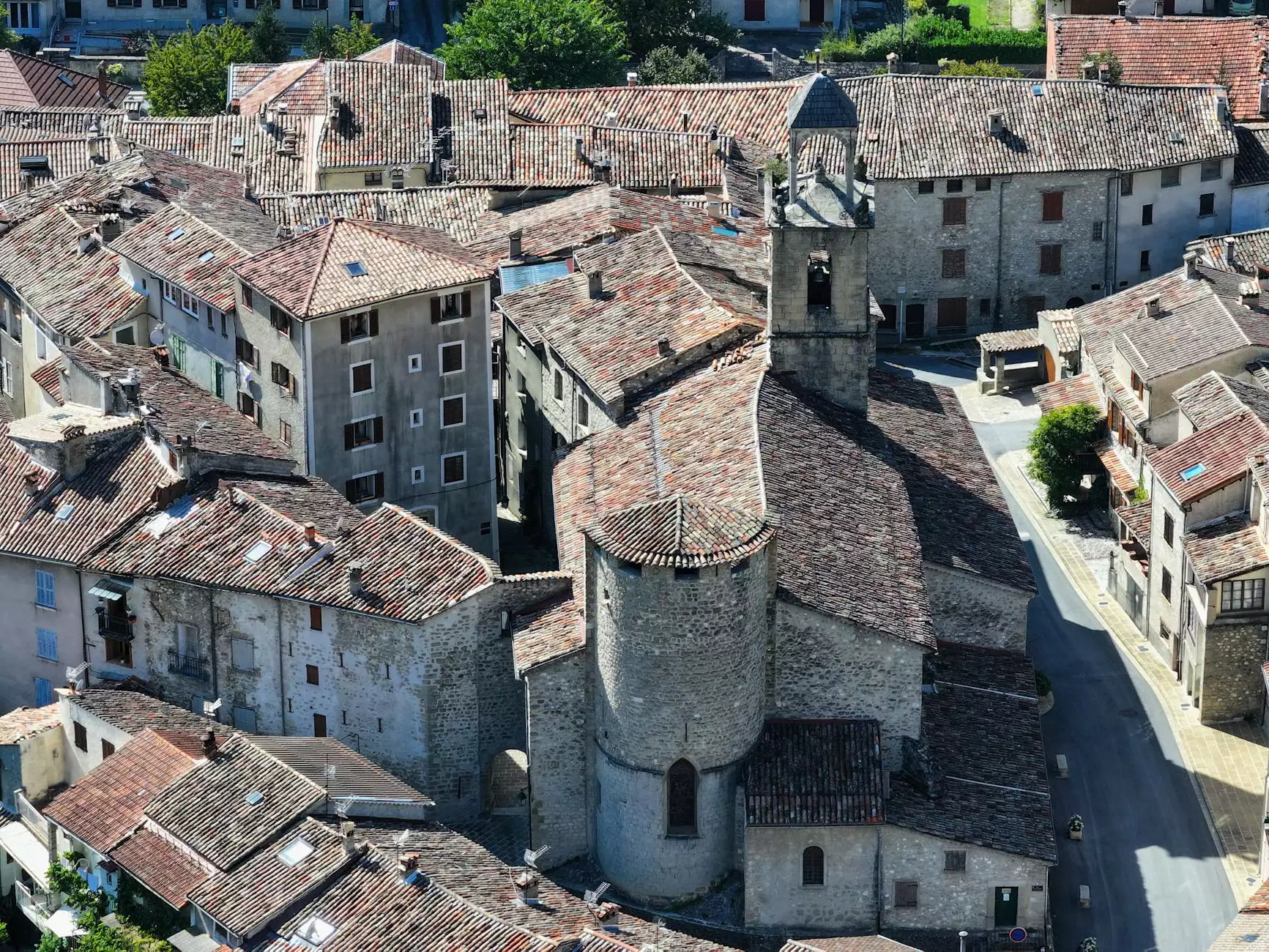
The Importance of Data Annotation in Machine Learning
In the realm of machine learning, data is the foundational pillar upon which algorithms are built. However, raw data in its unstructured form lacks clarity and contextual meaning. This is where data annotation plays a crucial role. Annotated data enables machine learning models to recognize patterns, comprehend context, and ultimately drive decisions.
Labeling tools for machine learning significantly enhance the efficiency and accuracy of this annotation process. By utilizing advanced labeling tools, businesses can streamline their data preparation workflows, ensuring that their AI systems are trained on high-quality, relevant data.
Understanding Labeling Tools for Machine Learning
Labeling tools are specialized software applications designed to assist in the data annotation process. These tools enable users to categorize, tag, and clarify data across various formats, including images, videos, and text.
Key Features of Effective Labeling Tools
- User-Friendly Interface: A well-designed, intuitive interface helps reduce the learning curve for annotators, allowing them to focus on the task rather than the tool itself.
- Collaboration Capabilities: Features that support collaboration among multiple users enable teams to work together efficiently, sharing insights and feedback in real time.
- Batch Processing: The ability to annotate multiple datasets at once accelerates the overall annotation process—saving time and resources.
- Customizability: Powerful labeling tools offer customization options for different data types and specific project needs, ensuring a tailored workflow.
- Quality Assurance Mechanisms: Integrated quality checks help maintain high standards, ensuring that annotated data meets the necessary criteria for effective machine learning.
Types of Labeling Tools for Machine Learning
Various types of labeling tools exist, each catering to different data types. Understanding these tools is essential in selecting the right one for your needs.
1. Image Annotation Tools
These tools are designed to annotate images through techniques such as bounding boxes, polygons, and segmentation. Key labs utilize advanced image annotation tools to improve object detection and recognition tasks.
2. Video Annotation Tools
Video annotation tools allow for frame-by-frame tagging of video content, crucial for tasks like activity recognition and object tracking. These tools help in training models that require temporal awareness.
3. Text Annotation Tools
Text annotation tools focus on categorizing text data, including named entity recognition and sentiment analysis. They are invaluable for natural language processing applications.
4. Audio Annotation Tools
These tools enable the labeling of audio data, essential for applications in speech recognition and sound classification.
Keylabs.ai: Leading the Charge in Data Annotation Platform
Keylabs.ai stands out in the crowded space of data annotation platforms. By focusing on both innovative technology and user experience, Keylabs.ai has developed a suite of labeling tools that transform the data annotation landscape.
Why Choose Keylabs.ai?
- Advanced AI Integration: Keylabs.ai incorporates AI-driven functionalities that enhance data annotation processes, making them faster and more efficient.
- Comprehensive Toolset: From image and video to audio and text annotation, Keylabs.ai offers a complete toolbox for all your data annotation needs.
- Scalability: Whether you are a start-up or a large enterprise, Keylabs.ai can scale to meet your project needs without compromising on quality.
- Robust Security Features: Understanding the importance of data security, Keylabs.ai employs industry-standard practices to protect user data throughout the annotation process.
- Exceptional Support: With a dedicated customer service team, Keylabs.ai ensures that users receive the help they need, whenever they need it.
Challenges in Data Annotation and How to Overcome Them
Despite the significance of data annotation, several challenges can hinder the process:
1. Time Constraints
As datasets grow exponentially, annotating them can become time-consuming. To overcome this, adopting efficient labeling tools for machine learning can accelerate the process, allowing teams to focus on analysis rather than manual tagging.
2. Ensuring Quality and Consistency
Maintaining consistency across annotated data is crucial. Implementing automated quality checks within the annotation process can significantly reduce errors and improve the reliability of the training data.
3. Managing Diverse Data Types
Organizations often deal with a variety of data types. Using a unified data annotation platform like Keylabs.ai, which supports dual functionalities—labeling tools for numerous data types—can simplify management.
The Future of Data Annotation in Machine Learning
As machine learning continues to evolve, the role of data annotation will become increasingly pivotal. Organizations will increasingly rely on automated labeling tools enhanced by artificial intelligence to improve the accuracy and speed of data preparation. The emergence of new techniques, such as weak supervision and semi-supervised learning, will further alter how data is annotated and consumed by algorithms.
Investing in cutting-edge labeling tools will be essential for companies seeking a competitive edge in the marketplace. As the tools become more sophisticated, the capacity for creating robust AI will increase, opening new avenues for innovation and growth.
Conclusion
In conclusion, the advent of labeling tools for machine learning marks a significant advancement in the efficiency and quality of data annotation. Utilizing platforms like Keylabs.ai can empower organizations to harness their data effectively, bridging the gap between raw input and actionable insights. As the landscape of machine learning continues to shift, businesses that prioritize effective data annotation will pave the way for future innovations and breakthroughs.