The Power of Machine Learning in Email Spam Filters
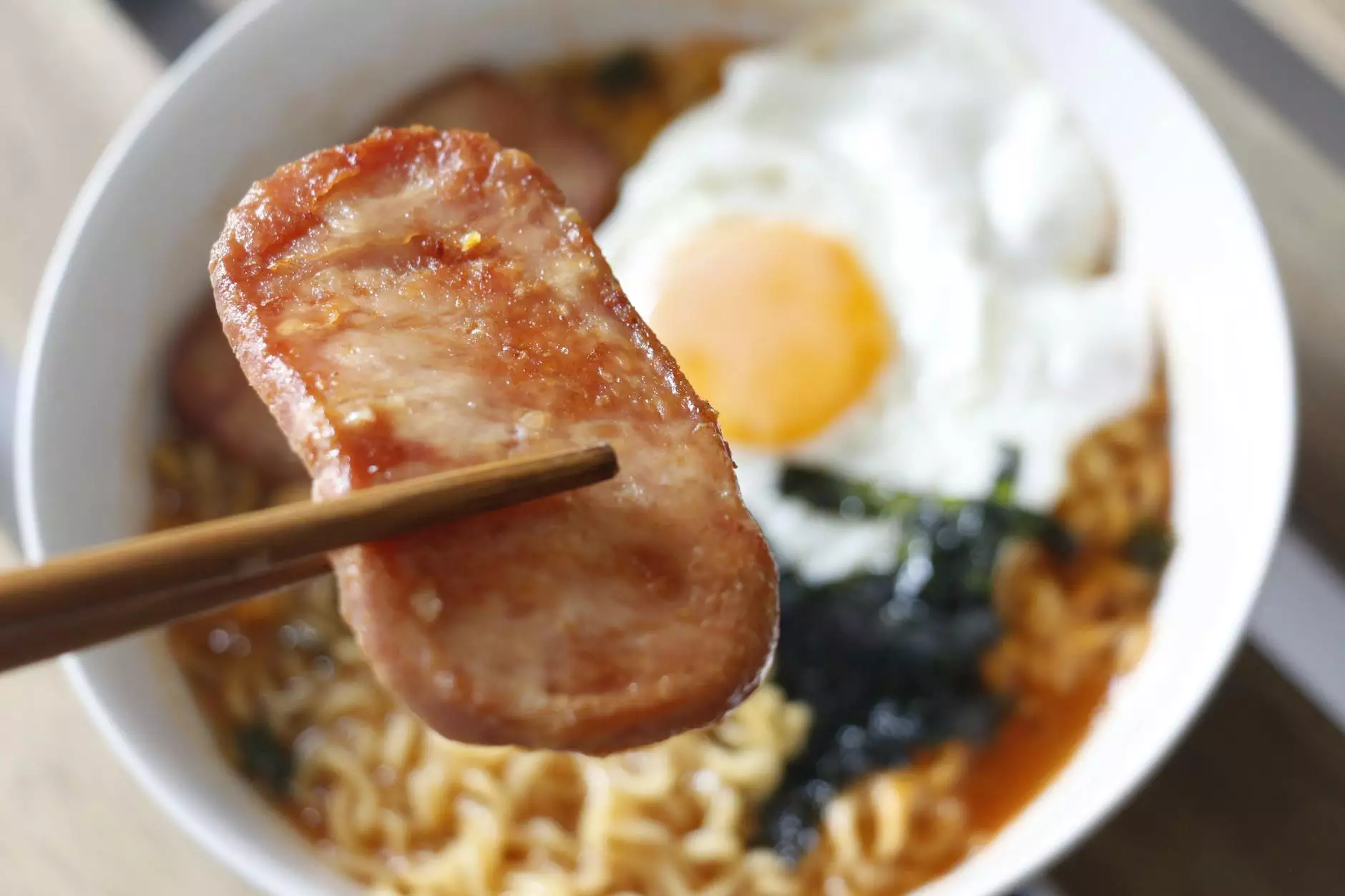
The digital age has brought about a tremendous increase in the volume of emails sent and received daily. Amidst this ever-growing flow of information, ensuring that your inbox remains free of unwanted spam is crucial. This is where email spam filter machine learning comes into play. In this article, we explore the intricate world of machine learning in spam filtering, how it operates, its advantages, and why it is essential for businesses and individuals alike.
Understanding Email Spam Filtering
Email spam filtering is a crucial process in digital communication. Spam, or unsolicited emails, can disrupt productivity, waste time, and pose significant security risks. Efficient spam filtering systems utilize various techniques to identify and classify spam emails, thereby protecting users from potential threats. However, as spammers develop more sophisticated methods, traditional filtering techniques are no longer enough. This is where machine learning (ML) plays a pivotal role.
What is Machine Learning?
Machine learning is a branch of artificial intelligence (AI) that focuses on the development of algorithms that enable computers to learn from and make predictions based on data. In the context of email spam filtering, machine learning algorithms analyze patterns in email data to distinguish between legitimate messages and spam. The beauty of machine learning is its ability to improve over time, learning from new data to enhance accuracy.
How Machine Learning Enhances Email Spam Filtering
1. Improved Accuracy
Traditional spam filters often rely on rule-based systems that require constant updates to address new spam tactics. In contrast, machine learning models can analyze vast amounts of data and learn from them, continuously improving their ability to predict spam.
- Pattern Recognition: Machine learning algorithms can identify complex patterns and correlations that are often missed by manual filters.
- Adaptive Learning: These systems can adapt to new threats by analyzing incoming emails in real-time, leading to improved filtering accuracy.
2. Reduced False Positives
One of the major challenges with traditional spam filters is that they can mistakenly classify legitimate emails as spam (false positives). Machine learning minimizes this risk by utilizing historical data to understand user behavior and preferences, resulting in fewer legitimate emails being misclassified.
3. Real-Time Processing
Machine learning models can process emails in real-time, analyzing the content and sender's history almost immediately. This speed is essential in today's fast-paced business environments where timely communication is key.
Techniques Used in Machine Learning for Spam Filtering
1. Supervised Learning
In supervised learning, algorithms are trained using labeled datasets where emails are classified as "spam" or "not spam." Over time, the system learns from this data, improving its prediction capabilities.
2. Unsupervised Learning
This approach does not require labeled datasets. Instead, the algorithm identifies patterns and clusters in data itself. Techniques such as clustering and association are used, providing greater flexibility in discovering new types of spam.
3. Neural Networks
Neural networks, specifically deep learning models, have shown remarkable success in spam filtering. These models simulate human brain processing, enabling the analysis of complex email attributes to discern spam from legitimate content.
Implementing Machine Learning in Email Spam Filters
1. Data Collection
The effectiveness of machine learning in email spam filtering hinges on the quality and quantity of data available. Businesses must collect data from various sources, including:
- Email headers
- Text content of emails
- User feedback on spam classification
2. Training the Model
Once data has been collected, it must be preprocessed and divided into training and testing sets. The model is trained on the training set to identify spam characteristics accurately.
3. Continuous Learning and Updating
Spam filters should be regularly updated with new data to adapt to evolving spam tactics. Continuous learning mechanisms ensure that the model remains effective against new threats.
Challenges of Using Machine Learning in Email Spam Filtering
1. Data Privacy Concerns
With increasing scrutiny over data privacy, businesses must ensure that user data is handled responsibly and ethically. Compliance with regulations like GDPR is essential when implementing machine learning solutions.
2. Resource Intensive
Machine learning requires significant computational resources. Small businesses may find it challenging to invest in the necessary hardware and software for effective spam filtering.
3. Dependence on Data Quality
The success of machine learning algorithms relies heavily on the quality of data used for training. Inaccurate or biased data can lead to ineffective filtering and increased false positives or negatives.
Benefits of Email Spam Filters Utilizing Machine Learning
1. Enhanced Security
Machine learning-powered spam filters significantly reduce the likelihood of phishing attacks and other malicious threats, which are common in unsolicited emails, thus enhancing overall security for users and organizations.
2. Improved User Experience
A cleaner inbox leads to better productivity. With effective spam filters in place, users can focus on important messages without the distraction of irrelevant spam emails.
3. Cost Efficiency
By automating spam detection and reducing the time spent managing unwanted emails, businesses can save on operational costs and allocate resources more effectively.
Best Practices for Businesses Implementing Machine Learning Email Spam Filters
1. Choose the Right Algorithms
Different algorithms may yield varying results based on the data being processed. It’s essential to experiment with different machine learning models to find the most effective for specific email scenarios.
2. Regularly Update Datasets
Spam tactics evolve rapidly. Businesses must ensure regular updates to their datasets for better accuracy and predictions.
3. Monitor and Analyze System Performance
Continuously tracking the performance of spam filters enables businesses to make necessary adjustments, ensuring that the system operates at peak efficiency.
Future of Email Spam Filters with Machine Learning
The landscape of email communication is changing. With the incorporation of advanced machine learning technologies, the future of email spam filtering holds promising developments:
- Predictive Analytics: Enhanced models will predict spam trends using historical data, allowing preemptive responses.
- User-Centric Filtering: Systems will become more tailored to individual users, improving accuracy based on personalized learning.
- Integration with Other Security Systems: Machine learning spam filters will likely work in tandem with broader security systems, providing holistic protection.
Conclusion
The role of email spam filter machine learning can’t be overstated in today's digital environment. As businesses continue to face the challenges of spam and cybersecurity threats, the implementation of machine learning models offers a sophisticated solution. By harnessing the power of data, organizations can enhance their email communication security, improve user experience, and foster an efficient work environment.
For companies looking to improve their email security, consider implementing advanced spam filtering solutions, like those provided by Spambrella. With a commitment to utilizing cutting-edge technology, Spambrella offers innovative IT services and computer repair, alongside state-of-the-art security systems designed to protect your digital communications.
Take Action Now
Don't let spam compromise your productivity or security. Explore machine learning-enhanced email spam filtering solutions today and experience the benefits of a cleaner inbox and a safer digital environment.