The Ultimate Guide to Data Annotation Tools and Platforms
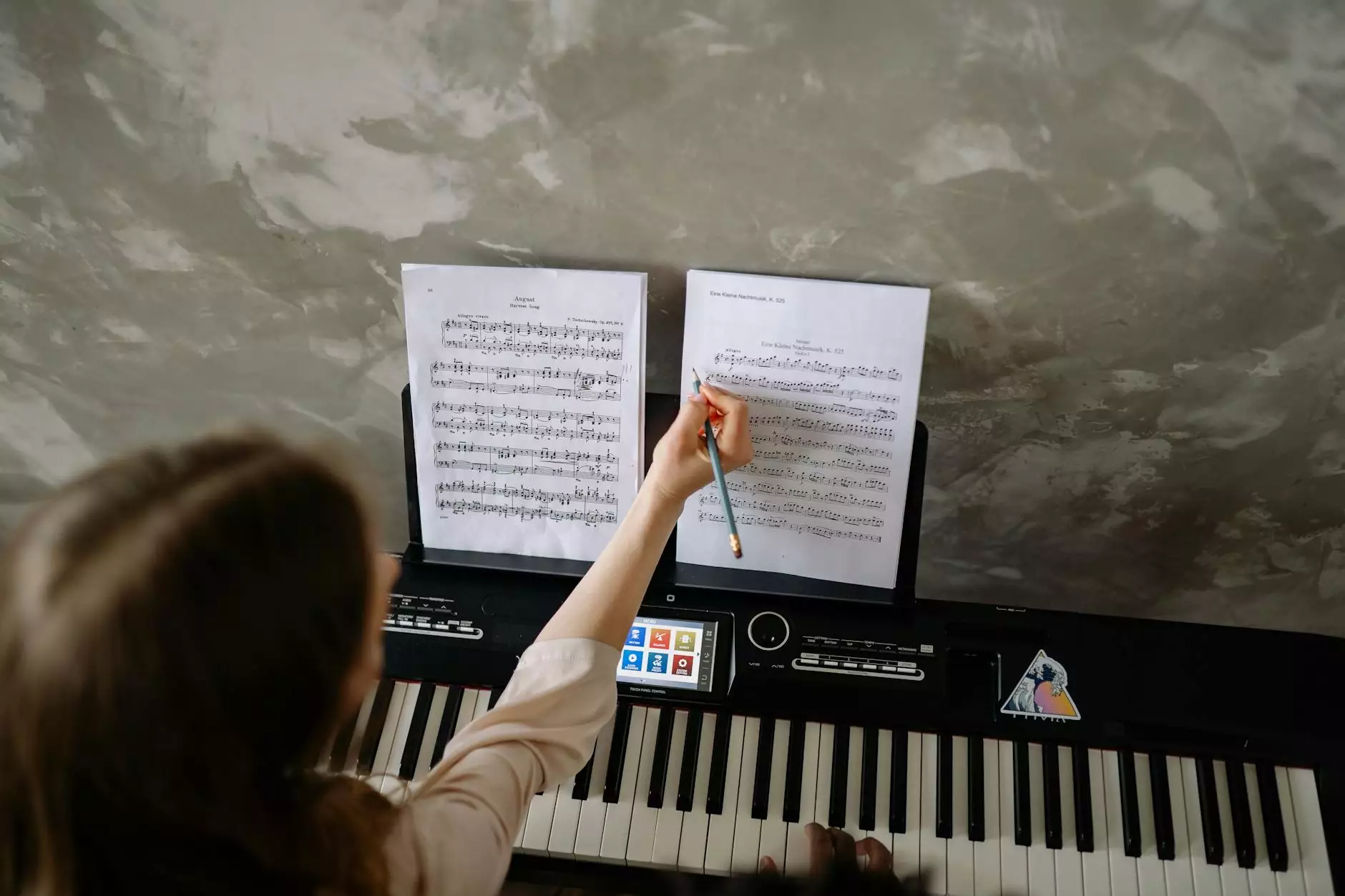
In today's fast-paced digital landscape, businesses are increasingly reliant on data to drive their decision-making processes and strategies. Data is the backbone of machine learning and artificial intelligence, and the effective annotation of this data is crucial for building efficient and reliable models. The importance of data annotation tools and platforms cannot be overstated, especially as we delve deeper into the era of big data. In this article, we will explore the significance of data annotation, the functionalities of the best tools available, and how https://keylabs.ai/ is setting a new standard in this domain.
Understanding Data Annotation
Data annotation refers to the process of labeling data to provide context, making it understandable for machine learning algorithms. This process is integral for supervised learning models which require labeled datasets to learn and make predictions accurately.
What is Data Annotation?
Data annotation can include a variety of data types such as:
- Image Annotation: Labeling images, identifying objects, and classifying scenes.
- Text Annotation: Tagging parts of text and categorizing written content.
- Audio Annotation: Labeling audio files, identifying speakers, and categorizing sounds.
- Video Annotation: Identifying objects within video frames and tracking movements.
Why is Data Annotation Important?
The effectiveness of machine learning models hinges on the quality of data they are trained on. Accurate and comprehensive data labeling ensures that algorithms learn effectively and perform better in real-world applications. Furthermore, data annotation helps in:
- Enhancing model accuracy.
- Facilitating quicker training times.
- Allowing for better predictions.
- Improving user experience across various applications.
Types of Data Annotation Tools
There is a plethora of data annotation tools available in the market, each designed to cater to specific needs and types of data. Understanding the types can help businesses choose the right tool for their projects. Here are some of the main categories:
1. Manual Annotation Tools
Manual annotation tools require human intervention to label data. While time-consuming, they often yield high-quality annotations, particularly for complex data types that require nuanced understanding.
2. Automated Annotation Tools
These tools utilize machine learning algorithms to automate the annotation process. They are particularly beneficial for handling large datasets quickly, although they may require initial human training to enhance their accuracy.
3. Semi-Automated Annotation Tools
Semi-automated tools combine both human input and automation, allowing for efficient processing while ensuring quality control through oversight by skilled annotators.
4. Specialized Annotation Tools
These tools focus on specific data types or industries. For example, tools designed for medical image annotation or those tailored for autonomous vehicle training datasets. Such tools often possess unique features that cater to particular use cases.
Key Features to Look for in a Data Annotation Tool
Choosing the right data annotation tool can be a daunting task. Here are several features to consider:
- User-Friendly Interface: A simple and intuitive interface helps in faster onboarding and easier operation.
- Collaboration Support: Tools that allow multiple users to annotate simultaneously can speed up the process significantly.
- Quality Control Options: Features that enable auditing or reviewing annotations can greatly enhance data quality.
- Integration Capabilities: The ability to seamlessly integrate with existing workflows and other software solutions.
- Scalability: As businesses grow, their data needs expand. A scalable tool can adapt to increasing data volumes.
The Role of Data Annotation Platforms
Data annotation platforms are comprehensive environments that house various tools and services for data labeling. They can support numerous annotation tasks, manage data workflows, and integrate with machine learning frameworks. Such platforms often provide advanced features including:
1. Robust Project Management
Good platforms enable users to manage multiple projects and datasets simultaneously, tracking progress and deadlines effectively.
2. Advanced Annotation Capabilities
Support for diverse annotation tasks across images, videos, text, and audio ensures versatility in usage.
3. High-Quality Output
Dedicated platforms for annotation often employ advanced algorithms to enhance output quality, leading to stronger model training.
How KeyLabs Redefines Data Annotation
https://keylabs.ai/ stands out in the realm of data annotation by providing a cutting-edge platform that addresses the complexities and demands of modern businesses. Here are key elements that make KeyLabs unique:
1. User-Centric Design
KeyLabs is designed with the user experience in mind. The intuitive layout and easy navigation facilitate quick setup and seamless operation.
2. Comprehensive Annotation Tools
With capabilities ranging from image and text annotation to audio and video labeling, KeyLabs provides an all-in-one solution that meets diverse project needs.
3. AI-Powered Automation
The advanced AI algorithms underpinning KeyLabs' platform allow for rapid data processing and annotation, increasing throughput while maintaining accuracy.
4. Collaborative Framework
KeyLabs encourages collaboration among team members, enabling them to work on projects together in real time, which streamlines the annotation workflow.
Best Practices for Data Annotation
To maximize the effectiveness of your data annotation efforts, consider the following best practices:
- Define Clear Guidelines: Provide annotators with detailed instructions to minimize errors and achieve consistency.
- Utilize Multiple Annotators: Use multiple annotators to label the same data and reconcile differences to enhance quality.
- Regularly Review Annotations: Establish a routine for reviewing and auditing annotations to maintain high standards.
- Leverage Technology: Use data annotation tools like KeyLabs to enhance productivity and quality.
Future Trends in Data Annotation
As technology evolves, so will the landscape of data annotation. Here are some predictions for the future of this critical domain:
1. Increased Automation
With advancements in AI and machine learning, we can expect tools to become increasingly autonomous, reducing the need for human intervention in repetitive tasks.
2. Enhanced Integration with AI
Data annotation platforms will likely integrate more closely with machine learning models, allowing for real-time training and feedback loops.
3. Focus on Quality Assurance
As the demand for high-quality data increases, tools will include more sophisticated quality assurance mechanisms to ensure data integrity.
Conclusion
In conclusion, data annotation tools and platforms play a pivotal role in the realm of machine learning, driving efficiency and enhancing the quality of models deployed across industries. With the capabilities provided by platforms such as KeyLabs, businesses can ensure they remain competitive in a data-driven world. Embracing best practices and staying ahead of emerging trends will be essential for organizations looking to leverage data annotation effectively. The future is bright for businesses ready to harness the power of annotated data!