Understanding Medical Data Annotation: Importance and Applications
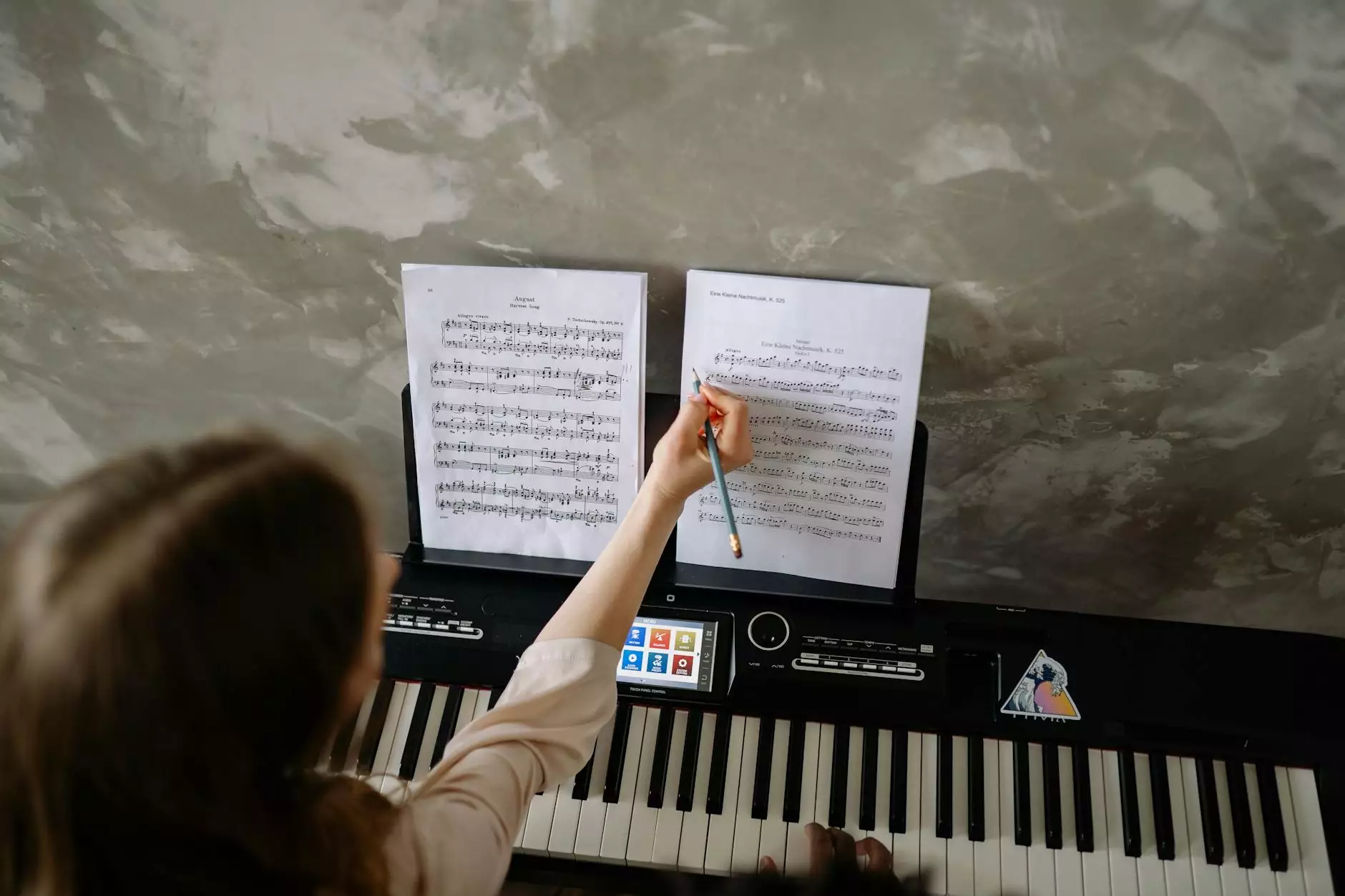
The healthcare industry is on the brink of a digital revolution, and at the heart of this transformation is the concept of medical data annotation. This process involves labeling data—such as images, texts, or videos—so that artificial intelligence (AI) and machine learning algorithms can effectively utilize it. The significance of this practice cannot be overstated, as it directly impacts patient care, diagnostic accuracy, and overall healthcare efficiency.
What is Medical Data Annotation?
Medical data annotation is the research and application of labeling medical data to create structured, usable datasets. In essence, it is the process of identifying and tagging relevant medical information in various formats. It is essential for training AI models in healthcare, which can analyze vast amounts of data to uncover patterns and insights.
Why is Medical Data Annotation Crucial?
The importance of medical data annotation can be abundantly highlighted by considering the following aspects:
- Improved Diagnostic Tools: Annotated medical images and texts are critical for developing diagnostic algorithms that can identify diseases and conditions more accurately.
- Enhanced Research: Researchers depend on annotated datasets to explore new treatment options and understand diseases better, facilitating groundbreaking research in various medical fields.
- Patient Safety: Accurate annotations help in creating tools that minimize diagnostic errors, which are crucial for patient safety and care outcomes.
- Efficiency in Healthcare Delivery: With annotated data, healthcare professionals can quickly access and interpret information leading to faster decision-making and improved patient throughput.
Types of Medical Data that Require Annotation
Medical data can come in various formats, and each requires specific annotation techniques. Some of the most common types of medical data that need annotation include:
1. Medical Imaging
Medical imaging is one of the most critical components of healthcare and includes:
- X-rays: Images that require annotation to identify fractures, tumors, or other conditions.
- MRIs and CT Scans: Detailed imaging requiring precise annotation to improve interpretation by radiologists.
- Ultrasounds: Often used in obstetrics, where annotated images can aid in tracking fetal development.
2. Electronic Health Records (EHR)
EHRs contain a wealth of information about patient histories, medications, allergies, and lab results. Proper annotation helps:
- Document clinical findings clearly.
- Facilitate better data sharing between healthcare providers.
- Enhance the quality of decision-making processes.
3. Clinical Trial Data
During clinical trials, vast amounts of data are generated. Annotated data can help:
- Streamline the analysis of trial outcomes.
- Ensure the data is categorized correctly for regulatory submissions.
Methods of Medical Data Annotation
The methods used in medical data annotation vary based on the type of data being annotated and the desired outcome. Here are some common annotation techniques:
1. Image Annotation
This involves tagging images with specific labels that reflect the data present in them. Techniques include:
- Bounding Boxes: Drawing boxes around specific objects in an image to indicate areas of interest.
- Segmentation: Dividing an image into its constituent parts for detailed analysis, crucial in identifying diseased tissues.
- Keypoint Annotation: Marking vertices of structures to capture their critical features.
2. Text Annotation
Involves labeling medical texts with categories or specific terms. Methods include:
- Named Entity Recognition: Identifying and classifying key terms (e.g., drugs, diseases) within the text.
- Contextual Tagging: Providing context for conditions or treatments mentioned in clinical notes.
The Impact of Medical Data Annotation on AI Technologies
The integration of AI in healthcare is revolutionizing patient care and clinical processes. However, AI models require robust, annotated datasets to learn effectively. Here’s how medical data annotation improves AI technologies:
- Training Robust Models: Annotated datasets allow machine learning models to learn and adapt to various medical conditions and patient histories.
- Reducing Bias: Diverse, annotated datasets help in training algorithms that are fair and unbiased in their predictions.
- Enabling Predictions: Well-annotated data empowers AI systems to make predictions, aiding healthcare professionals in clinical decision-making.
Future of Medical Data Annotation
The future of medical data annotation looks promising, with several trends indicating its expanding role in healthcare:
1. Increased Automation
As technology advances, we expect to see a greater reliance on computer-assisted annotation tools that utilize natural language processing (NLP) and image recognition.
2. Collaborative Annotation Platforms
The establishment of platforms that enable multiple professionals to annotate data collaboratively will likely increase the quality and volume of annotated datasets.
3. Enhanced Privacy Measures
As healthcare data privacy is paramount, innovative approaches will be developed to ensure that data is accurately annotated without compromising patient confidentiality.
Conclusion
Medical data annotation is not merely a technical necessity but a cornerstone of modern healthcare that enables innovations in diagnostics, treatment, and research. As the volumes of medical data continue to grow, so does the need for effective annotation methods to ensure that this data can be leveraged to improve patient outcomes. By investing in strategies and technologies for accurate medical data annotation, the healthcare industry can enhance overall efficiency, research capabilities, and ultimately, patient safety. Embracing this practice is essential for any healthcare provider looking to thrive in an increasingly digital and data-driven world.